Creating an MCP Server Using FastMCP: A Comprehensive Guide
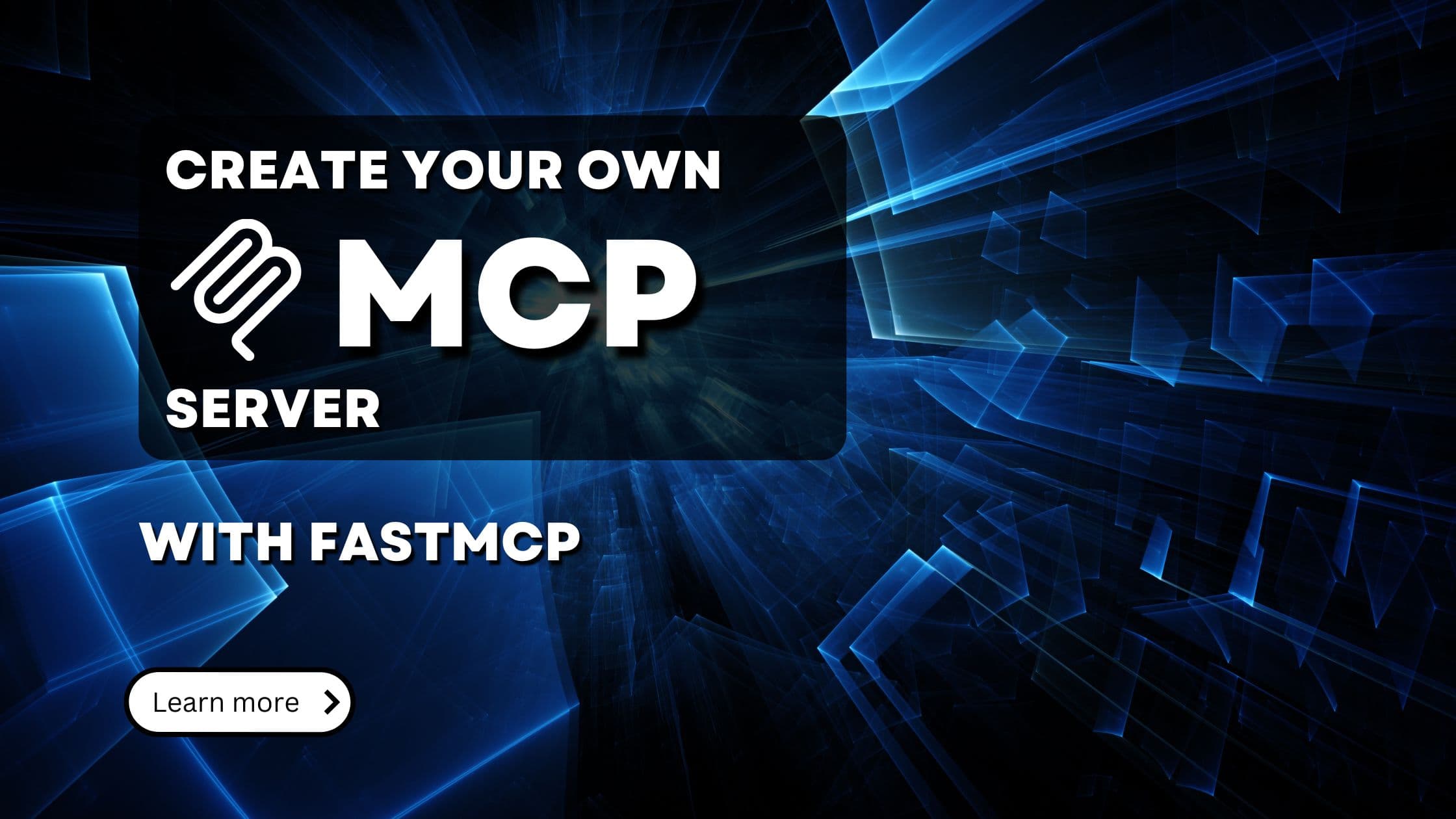
Building AI applications that can seamlessly access your data and tools is surprisingly challenging. The Model Context Protocol (MCP) solves this problem by standardizing how LLMs connect to external resources. Think of MCP like a USB-C port for AI applications - a universal connector that lets your language models plug into different data sources and tools with minimal friction.
FastMCP takes the powerful but complex MCP protocol and makes it accessible through a high-level, Pythonic interface. With just a few decorators, you can transform ordinary Python functions into MCP resources and tools that any MCP-compatible client (like Claude Desktop) can instantly use.
In this guide, we'll walk through creating your own MCP server using FastMCP, giving your AI assistants secure access to your data and custom functionality. Let's get started by building a simple server that can power your AI workflows.
What is an MCP Server?
An MCP server is a lightweight program that exposes your data and functionality to language models through the standardized Model Context Protocol. These servers act as bridges between AI applications (like Claude Desktop or other LLM-powered tools) and your local data sources or remote services.
The main thing to understand: MCP Servers are basically just a standardized API for accessing your internal tools or data.
At its core, an MCP server offers six main capabilities:
- Resources: Read-only endpoints that provide data to LLMs (similar to GET requests in web APIs)
- Tools: Functional endpoints that execute actions when called by an LLM (like POST/PUT endpoints)
- Prompts: Reusable templates that guide how LLMs interact with your server
- Sampling: The ability to request completions from the connected LLM. This basically means that if the server requires an LLM to complete it's own action (for example creating a summary), the server can request the client to execute the LLM completion (so that the client pays for the completion and can control which LLM is used (think of data privacy)).
- Transports: Communication mechanisms that enable clients and servers to exchange messages, such as stdio (Standard Input/Output) for local tools and SSE (Server-Sent Events) for web-based applications
- Roots: URI-based boundaries that define where servers can operate, allowing clients to inform servers about relevant resource locations (like file paths or API endpoints)
MCP servers operate within a client-server architecture where:
- Your MCP server runs locally or on your infrastructure
- MCP clients (like Claude Desktop) connect to your server
- Your server provides secure access to your files, databases, APIs, or custom functionality
Unlike traditional web APIs, MCP servers are specifically designed for LLM interactions, with features for natural language understanding, context management, and LLM-specific functionality baked in.
By creating an MCP server, you enable AI assistants to interact with your data and services while keeping your information secure and within your control. No need to upload sensitive documents to third-party services or rebuild integrations for each new AI tool.
Added benefit: As anyone and their mum currently crave for MCP server tools, your software will have a marketing advantage when being able to offer an MCP interface.
General MCP Server idea
MCP Server Example
If the above description is too abstract, let's discuss a simple example. Github offers an MCP Server, which in terms offers various tools to interact with Github, for example there are tools to get repository information or tools to create pull requests.
As many AI chat tools nowadays already provide an MCP client implementation, all these tools can now connect to Github and create AI-powered workflows. This is sort of a win-win situation: All these tools don't need to integrate the Github API (and as a matter of fact many, many other APIs), but they simply implement the MCP Client. As the MCP protocol allows LLMs to intelligently read the available tools, they automatically are aware about all the available tools ("API endpoints", if you will).
What's the difference between MCP servers and an API
As we already established, MCP servers - at their core - are just standardized APIs. So why not use the millions of existing APIs out there? The simple answer is: MCP servers make it easy for LLMs to discover what tools and resources are available in this API. Furthermore, they standardize authentication and transport. If enough people agree on MCP being the way forward (and currently it looks like many people agree that this will be the way forward, like Microsoft), Anthropic, Google and OpenAI), we got one single interface which is optimized for LLM discoverability. All client-side tools therefore only need to implement the MCP client, all the rest is handled by the protocol and the LLMs using the MCP client. Note that the MCP protocol in itself only really makes sense when you have LLMs which can "read" the provided resources/tools and then act upon them.
If you simply need to call APIs, ordinary REST endpoints are still the way to go.
One other further advantage of MCP servers: As the protocol seems to be widely adopted by AI client tools, you can also create a local MCP server to provide controlled and secure access to resources on your system. For example allow file-system access, but only on a certain file mount.
What is FastMCP?
FastMCP is a high-level Python framework that dramatically simplifies the process of building MCP servers. While the raw MCP protocol requires implementing server setup, protocol handlers, content types, and error management, FastMCP handles all these complex details for you.
At its heart, FastMCP provides a decorator-based API that transforms regular Python functions into MCP-compatible tools, resources, and prompts. The framework's key advantages include:
- Minimal Boilerplate: Create fully-functional MCP servers with just a few lines of code.
- Intuitive Design: The decorator pattern will feel familiar to developers who've used frameworks like Flask or FastAPI.
- Automatic Schema Generation: FastMCP uses type hints and docstrings to automatically generate the necessary MCP schemas without manual configuration.
- Pydantic Integration: Leverage Pydantic models for complex inputs and outputs with automatic validation.
- Async Support: Built-in support for both synchronous and asynchronous function handlers.
- Transport Abstraction: Work with various communication protocols (stdio, SSE, WebSockets) without changing your application code.
- Context Object: Access server capabilities, log information, report progress, and even request LLM sampling through an intuitive context object.
- Client Implementation: Includes a full client implementation for testing your servers programmatically.
FastMCP's primary goal is to make MCP development accessible to Python developers of all skill levels while still exposing the full power of the protocol. The framework handles all the low-level protocol details, allowing you to focus on implementing your business logic rather than wrestling with the complexities of the MCP specification.
With FastMCP, you can go from concept to working MCP server in minutes rather than hours, making it the ideal choice for rapidly prototyping AI-powered tools or building production-ready MCP services.
Great detail: FastMCP was so successful, that it got directly integrated in the official MCP Python SDK.
Hands on: Building an MCP server using FastMCP
Now that we know what we need to know, let's dive in. We're going to build a simple MCP server which allows to:
- Fetch a users profile (Resource)
- Update a document on the server (Tool)
- Add progress reporting (Context)
Prerequisites: Basic MCP Server setup
First, let's create a virtual environment and install fastmcp (this also contains the mcp dependencies).
Then, let's create the basic server instantiation:
This snipped will run an MCP server (without any resources yet) with STDIO transport mode. What does this mean:
- The client starts a new server process for each session
- Communication happens through standard input/output streams
- The server process terminates when the client disconnects
- This is ideal for integrations with local tools like Claude Desktop, where each conversation gets its own server instance
FastMCP Transports explained
Above, we created our first little MCP server (which doesn't do anything
yet). However, we learned that this server runs with STDIO transport.
This, for obvious reasons, means that this server is mainly used to
provide access to resources local to your system. While there are many use
cases for that, we think the main advantage of MCP servers lies in
providing a remote-accessible resource to allow controlled and secure
access to your internal systems. Therefore, FastMCP offers a second
transport: SSE Transport (Server Sent Events)
.
To use this transport, simple use:
This will spawn a server listening on all interfaces and port 8000.
Interesting to know: FastMCP in SSE transport mode uses Starlette for providing the ASGI interface and uvicorn to wrap it in a server.
We'll use this transport forward.
Creating an MCP Resource using FastMCP
Resources offer read-only access to internal data and information. Resources are requested by providing a URI (which if often AI generated on the client-side). The FastMCP uses this URI to find the requested resource, looks for the data somewhere in it's environment and then returns them to the client.
Defining a Resource
is rather simple.
-
Create a python function which returns the data you want your resource to return.
-
Add a fastmcp resource decorator with the unique URI of this resource as parameter.
-
Optionally add additional metadata, like description or tags.
Note: These additional metadata can be used by clients for filtering or deciding which resource to call. As with any LLM-based application these metadata are rather important that the (client)-AI knows what to do. So we mostly recommend always using all the meta-information.
These metadata are available:
- uri: The unique identifier for the resource (required).
- name: A human-readable name (defaults to function name).
- description: Explanation of the resource (defaults to docstring).
- mime_type: Specifies the content type (FastMCP often infers a default like text/plain or application/json, but explicit is better for non-text types).
- tags: A set of strings for categorization, potentially used by clients for filtering.
Important: Make sure your functions are async when using disk or remote operations, to not block the server.
Imporant: The MCP uses the doc-strings of your functions to generate a description for the LLM. Therefore it's important to use good, descriptive ones. Note that if you set the description in the decorator via parameter, the docstring is not needed (and is actually ignored).
To summarize what we have so far: By providing the uri
data://application-information
, a client can get the defined application
status report. So good so far. Now we want to implement returning a user
profile. The problem: We currently don't have the option to pass the user id
to the MCP server, right? As we only have the URI to exchange data.
This is where resource templates come into play.
MCP Resource templates
Resource templates are simply a way to tell the MCP server, that parts of the
URI path in the resource defintion is dynamic. Eg. we can define the following
URI to implement our user-profile resource: data://user-profile/{user_id}
.
The resource function than simply takes an additional parameter for the
dynamic value.
Perfect, now the clients simply use the resource alongside a dynamic user_id
parameter.
Creating an MCP Tool using FastMCP
Tools are, in short, the most important parts of any AI agent systems. Tools
are functions which allow the AI the "interact" with the external world.
Let's say you have a function which makes a database call. You transform this
function to a tool
, allowing the AI to call this function and therefore
access your database.
Note: When we talk about AI "calling" a function, technically, they don't call anything (as an AI model itself can't run code etc.). The AI model simply returns (via text), that it wants to call a specific tool (which are just python functions), and you execute this function on behalf of the AI.
For an in-depth introduction into what tools are, we highly recommend our AI Agents from scratch article.
Now, similarly to resources, with FastAPI we don't really need to care about the underlying protocol or who calls what. Just create our function, which executes something, add a decorator and optionally add some metadata parameter.
FastMCP:
- Uses the functions name as tool name. Make it descriptive.
- Uses the docstring (or the decorator description param) as tool description.
- Uses type-annotations for schema generation.
- Validates the incoming data based on this type schema.
Again, make sure you make your tools async, if they require waiting tasks.
Adding parameter metadata
Everyone who created AI agents (or clients) in the past knows, that the most important aspect of creating tools is actually the tool description, and more so the tool parameter description. So that the AI knows, which parameters to provide.
For that, FastMCP allows to use the pydantic
Annotated
field to add more
information to the parameters.
Furthermore, parameters with default values are communicated as optional.
Error handling with FastMCP tools
Now the final piece of the puzzle, how do we communicate errors with the AI? Most of the time you want to inform the AI that there was an error executing a tool, so that they can decide to run the tool differently or use a different one.
Luckily, this is very easy in FastMCP: Simply throw a standard python Exception, like we did above.
FastMCP converts the exception into an MCP error response, which is sent back to the client. The client can then decide what to do with the error, but mostly they will want to redirect it to the LLM for further action taking.
Supported types with FastMCP tools
For tool input parameters, FastMCP basically supports most of the types which are also supported by pydantic. This includes, but is not limited, to all built-in types, dates, literals, enums, collections (dicts, lists), uuids, complex pydantic models and many more. For a complete list, please visit the FastMCP docu
For output types, we are a bit more limited. These are the supported output (return) types:
- str: Sent as TextContent.
- dict, list, Pydantic BaseModel: Serialized to a JSON string and sent as TextContent.
- bytes: Base64 encoded and sent as BlobResourceContents (often within an EmbeddedResource).
- fastmcp.Image: A helper class for easily returning image data. Sent as ImageContent.
- None: Results in an empty response (no content is sent back to the client).
Implement MCP server progress reporting using FastMCP Context
The FastMCP Context allows to access the underlying MCP session or to access your MCP servers capabilities. Examples for that are:
- Logging: Send log messages from your MCP server back to the client.
- Accessing Resources: In your MCP server tools, you might want to access already defined resources. Eg. get the file-path from a resource.
- Progress reporting: Send progress update to the client for long-running operations.
There are more advanced context options available, which are not in scope for this article. Feel free to read up on them here. We'll also provide an article about them in the near future.
For our small project, we are going to implement progress reporting, to showcase the Context feature.
Again, very simple to do:
-
To the function (resource or tool) where you want to access the context, simply add a parameter
ctx
of typeContext
(the name does not matter, the type-hintContext
needs to be provided though). -
Then simply use the
ctx.report_progress(progress, total)
method to send progress back to the client.
Note: Context methods are mostly async. So make sure to await them.
That's it.
Testing your MCP server
Now that we've created ourselves an MCP server, we need to run it. As outlined, FastMCP already packages uvicorn, so simply run your python file. If you followed this guide, the server will use the SSE transport and listen on port 8000.
Either use a tool like Claude Desktop or create a simple MCP client and test the capabilities.
For a fill reference of available client methods for the FastMCP client, see their documentation
Wrapping up
In this article we've built ourselves a fully-fledged MCP server, providing tools, resources and status reports to any connected clients. Using this server any client implementing the MCP protocol now can connect to our tool - basically offering a universal way for allowing AI clients to interact with our tools and servers.
Further reading
- Smolagents: Minimal Agent Framework: Explore a lightweight approach to agent development
- Langfuse: The Open Source Observability Platform: Monitor and improve your LLM applications
- Evaluate RAG Performance Using RAGAS: Learn how to measure and optimize your retrieval systems
- High-Quality AI Agent Systems: Best practices for building robust AI agents
Interested in how to train your very own Large Language Model?
We prepared a well-researched guide for how to use the latest advancements in Open Source technology to fine-tune your own LLM. This has many advantages like:
- Cost control
- Data privacy
- Excellent performance - adjusted specifically for your intended use